Principal Component Analysis explained visually
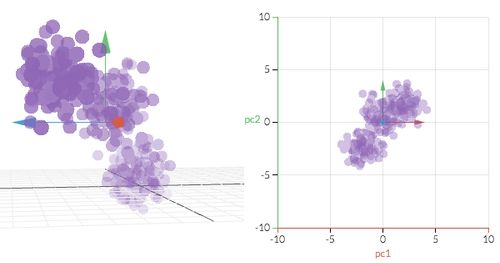
Principal Component Analysis explained visually
8/22/2021
link
summary
This webpage provides an interactive visualization and explanation of Principal Component Analysis (PCA). PCA is a statistical technique used to simplify high-dimensional data by identifying the most important features or variables. The article breaks down the steps involved in PCA, such as centering the data, calculating covariance matrix, and finding eigenvectors and eigenvalues. The interactive visualization allows users to experiment with different datasets and see how PCA transforms the data. It also provides a clear explanation of the underlying mathematics and concepts behind PCA, making it a useful resource for anyone looking to understand and apply this technique.
tags
principal component analysis ꞏ pca ꞏ data analysis ꞏ dimensionality reduction ꞏ linear algebra ꞏ statistical modeling ꞏ data visualization ꞏ exploratory data analysis ꞏ feature engineering ꞏ machine learning ꞏ data science ꞏ mathematical modeling ꞏ multivariate analysis ꞏ data preprocessing ꞏ pattern recognition ꞏ algorithm ꞏ data mining ꞏ unsupervised learning ꞏ eigenvalues ꞏ eigenvectors ꞏ variance ꞏ covariance ꞏ data compression ꞏ dimension reduction ꞏ data exploration ꞏ statistical analysis ꞏ data interpretation ꞏ data transformation ꞏ mathematical optimization ꞏ feature extraction ꞏ data representation ꞏ data reduction ꞏ data clustering ꞏ data patterns